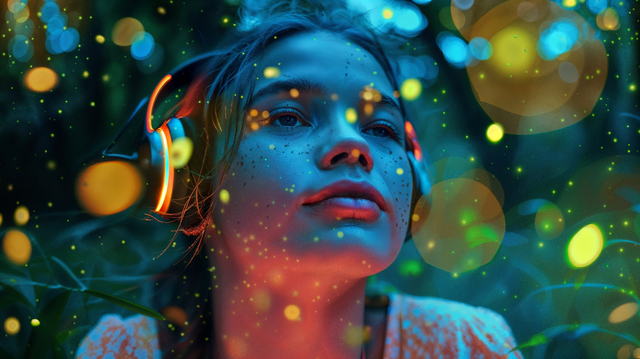
Singapore-based Bank Benefits from Groundbreaking Machine Learning Operations Platform
Singapore-based Bank Benefits from Groundbreaking Machine Learning Operations Platform
Please provide your details
Asterisk (*) indicates required field.
Thank you!
Your submission has been forwarded to the appropriate contact.
Challenge: Slow, Admin-heavy Processes Obstruct ML at Scale
A Singapore-based digital bank looking to power customer-centric growth with ML was struggling to replicate early ML success at scale. Laborious processes surrounding the development, approval, and launch of ML models were at the root of the problem. In the financial services sector, technical complexity of ML is heightened by stringent security, compliance, and regulatory requirements. For this market, the Monetary Authority of Singapore (MAS) toolkit for responsible use of AI in the financial sector was another consideration.
When the bank wished to scale use of ML scaled to support high growth targets, its data scientists could not meet expectations since they became overburdened with low-value manual tasks that distracted them from the core job of developing, testing and launching ML models. To reduce friction and streamline processes, these tasks needed to be built into a workflow enhanced with modern tools. As cloud consultancy partner, Amdocs was engaged to help the bank achieve its ML vision. We harnessed MLOps capabilities, automating key aspects of the model lifecycle to maximize productivity, efficiency, and compliance, thereby supporting scalability.
Solution: Pioneering MLOps Platform Enables Fast, Consistent Production
Amdocs’ engineering experts built an MLOps platform leveraging Amazon SageMaker. The platform-based approach automated activities previously handled by the data scientists and has policies baked in to satisfy stringent financial services sector requirements.
The platform delivered ML model scalability and efficiencies, increasing the data scientist team’s productivity and delivering increased value to the organization.
Managed services from SageMaker provide scalable compute resources for model training and inference tasks. We used the auto scaling feature to ensure resources dynamically adjust based on workload demands, optimizing cost efficiency without sacrificing performance. Alongside this, we used Amazon EC2 Spot Instances for model training, taking advantage of cost savings while maintaining flexibility and reliability.
Additional measures were taken to enable efficient processing of large datasets, automated security and network controls, and standardized protocols for ML model observation. Together with work process automation, these capabilities streamline the ML model lifecycle, helping to secure stakeholder engagement and approval at critical points. They also reduce barriers to ML innovation, enhancing data scientists’ ability to experiment with and test models geared towards the enrichment of customer experiences.
This was the first banking sector MLOps platform built on AWS Singapore. Due to the pioneering nature of the project, Amdocs liaised closely with AWS during the onboarding, devising new processes where needed and sharing lessons learned.
Outcomes: Secure, scalable MLOps Boosts Data Scientist Productivity
The MLOps platform facilitates robust, frictionless processes which accelerate the development-to-production journey for ML models. Compliance with organizational and industry standards requires less human intervention, which has led to a two-fold improvement in productivity. Standardized approaches have also helped streamline the control of operational procedures. In short, the platform makes life easier for data scientists. It also empowers them to deliver organizational value though ML models that drive sustainable, customer-centric growth.
Implementing the Amdocs MLOps solution delivered the following results:
- Reduced onboarding time for new data scientists from months to days
- Reduced the time to bring a ML model from Development to Production from 3 months to 6 weeks
- Reduced time to respond to security events from hours to minutes
- Shortened response time to high spend events from days to hours