As financial services institutions (FSIs) explore the transformative potential of Generative AI, they face challenges in realizing its benefits. The technology serves to revolutionize customer experiences and drive innovation, but its success hinges on one critical factor: quality engineering.
Remove quality roadblocks to visionary AI
GenAI has the potential to deliver the high-touch, personalized experience typical of private banking to every customer. AI assistants will help people select and apply for loans faster. Retail investors will use natural language to learn more about the pluses and minuses of different retirement-saving strategies. An AI-powered coach will review your spending and highlight areas of interest for financial planning strategies, taking into account family dependents, obligations, and risk tolerance.
Depending on your perspective, next-generation financial applications powered by AI can seem right around the corner or years away. Naysayers point to quality considerations hampering financial services institutions' ability to realize visionary use cases. But what if quality isn’t the obstacle?
Instead, quality is the answer and the accelerant for real-world GenAI value. FSIs that are making quality engineering (QE) central to their AI strategies are surging ahead in the race to realize returns on GenAI. Our estimates reveal that FSIs leveraging the power of automated QE workflows are seeing near-term wins, including a 50% improvement in test coverage optimization, a 60% decrease in testing certification time, a 33% decrease in testing design time, and more.
Avoiding quality missteps
The excitement around GenAI for FSIs mirrors the early enthusiasm for cloud and DevOps transformations. While cloud and DevOps eventually lowered costs and accelerated processes for many organizations, early progress was often slowed by quality challenges, and much of their full potential is still unrealized.
Quality processes designed for on-premise systems proved ill-suited to cloud infrastructure. Testing at the end of the development process ate many of the speed gains of DevOps. As a result, FSIs have started to adopt quality strategies that are better suited to taking advantage of cloud and DevOps. Unfortunately, that adaptation has taken years for many FSIs. The adaptation to AI is still in its early stages, but it’s critical for FSIs to start their GenAI journeys with an effective quality strategy.
Developer and QE roles evolving fast
Consider how AI is already transforming developer and quality engineering roles. As more developers use GenAI for routine tasks, they will have more time for higher-level activities such as architectural design, enhancing the user experience, and integrating emerging technologies like GenAI into the customer experience.
Yet, AI-generated code places new speed and rigor demands on quality processes, elevating QE’s importance. QE specialists must play a strategic role in planning GenAI-powered software delivery. Otherwise, quality considerations will either reduce AI's productivity benefits or introduce levels of risk that are unacceptable to most FSIs. Forward-looking FSIs are getting ahead of development and QE evolution by increasing QE’s influence on GenAI adoption plans.
Connect GenAI implementation to QE
With GenAI boosting developer productivity by as much as 50%, the pace of development and innovation is changing. This speed advantage leads to a competitive advantage for FSIs that can harness GenAI to accelerate time to market for customer-focused features and services. Yet, as developers who use AI know, bot-generated code can contain errors and introduce vulnerabilities. FSIs must ensure that AI-powered development adheres to regulatory requirements and security standards while delivering a flawless customer experience.
The answer is to integrate QE into GenAI processes from the start, with continuous testing that leverages GenAI. In the first phase, machine learning helps automate testing at a speed that matches AI-powered development. It also improves precision in test selection, boosting overall efficiency.
Already, FSIs can leverage GenAI to create synthetic datasets that closely resemble real-world financial transactions while maintaining compliance with privacy regulations. With this on-demand synthetic data, you can rapidly scale testing for targeted use cases. Additionally, GenAI can be used to train AI agents to automatically generate test plans and test cases tailored to specific features and services, while ensuring alignment with regulatory requirements. Your quality engineers can use such agentic agents to augment their efforts, enabling them to conduct more efficient and intelligent testing of GenAI-driven innovations to benefit both customers and employees.
Grow AI and QE excellence on a solid foundation
How do you start enhancing GenAI with QE and vice versa? FSIs that incorporate real-world AI value into QE workflows today will be better positioned to deploy market-leading, customer-facing use cases in the future. It starts by adopting a maturity model for GenAI QE and targeting baseline maturity as soon as possible.
Baseline maturity includes GenAI-assisted QE. Here, GenAI serves as a knowledge base and guide for testers. Machine learning models help to optimize automated testing and provide training. The natural language capabilities of GenAI increase efficiency for QE specialists and deliver rapid insight into patterns and anomalies.
Starting now with baseline maturity as a goal positions FSIs to move faster toward greater AI autonomy and QE efficiency. The next maturity level — augmented GenAI QE — is already possible and opens the door to automated test design and planning. From there, agentic GenAI QE represents the next transformative step, introducing innovations like autonomous testing agents and self-optimizing testing. Many FSIs are already connecting AI and machine learning to automated QE workflows, and those who delay risk being left behind.
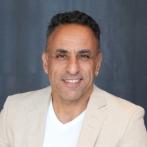
Dror Avrilingi - VP, Head of QE, GenAI & Data Studios
Dror is responsible for leading innovation in data and GenAI, and spearheading initiatives that transform how organizations utilize data to drive value. As part of this role, he drives the rapid expansion of GenAI use cases, advancing the ways data is leveraged to deliver actionable insights.