Addressing cell outages in near-real-time, particularly within a one-second window, necessitates a sophisticated approach. ML techniques are ideally suited to meet this challenge. The key is to train the system in advance using simulations that closely mimic real network conditions.
The effectiveness of Amdocs Cell Outage Compensation within the O-RAN near-real-time control loop and framework, demonstrating its potential to adapt and respond to the challenges presented by today’s complex network environments.
Addressing uncontrolled cell outages is key to preserving Quality of Service (QoS) in mobile networks, playing a crucial role in ensuring network coverage and enhancing capacity under the dynamic conditions of modern network operations.
Our findings affirm that reinforcement learning is a fitting method for compensating for cell outages quickly and efficiently, allowing the network to adapt seamlessly when a cell becomes unavailable or is restored.
Get the whitepaper
Thank you for your interest!
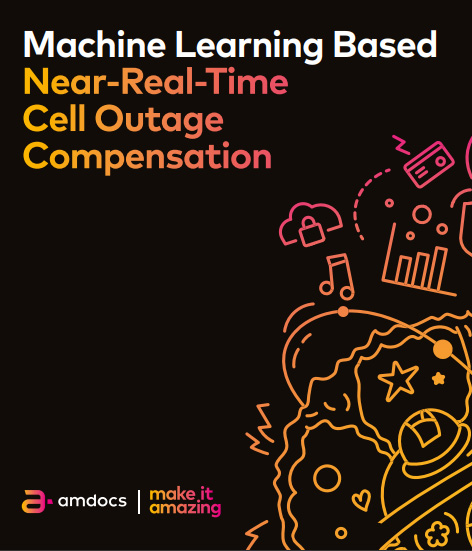