Test new configurations, optimize performance, and deploy seamlessly. AI-powered digital twins create a dynamic sandbox for autonomous networks, enabling predictive analytics, real-time decision-making, and continuous optimization. Discover how GenAI and intelligent agents transform network management into a fully automated, self-optimizing ecosystem.
How AI-powered digital twins are driving autonomous telco networks
Imagine testing radical network changes without risking a single dropped call or service disruption. That’s the power of the digital twin “sandbox” for autonomous networks (ANs). While ANs promise self-managing, self-optimizing telecom networks, their complexity demands a safe testing ground. Digital twins, virtual replicas of real-world networks, provide precisely that – a risk-free environment allowing experimentation with new configurations, simulating diverse scenarios, and validating algorithms before live deployment.
But what is it that truly transforms this sandbox into a dynamic, intelligent innovation hub? The answer lies in the integration of cutting-edge AI, with GenAI and advanced autonomous agents.
In the context of agentic AI, digital Twins, and ANs, an agent is an AI-driven entity that autonomously interacts with the digital twin, making decisions, learning from simulations, and optimizing network configurations. In the autonomous network ecosystem, these agents continuously analyze data, predict network behaviors, and adapt to evolving conditions, enhancing efficiency and resilience. By enabling independent decision-making and collaboration, agents transform digital twins from static models into dynamic, intelligent environments that drive real-world network innovation and automation.
A sandbox for autonomous innovation
The core value of a digital twin lies in its role as a safe and isolated "sandbox" – a complete virtual replica of the physical network, mirroring everything from hardware and software configurations to real-time data flows. There are several advantages – First, it provides complete isolation: changes have absolutely no impact on the live network. Second, it enables network scenarios to be replicated and analyzed repeatedly under identical conditions – from planned upgrades to complex service fulfillment processes. Third, it offers a controlled environment, where network operatives can manipulate variables like traffic loads, service and network orchestration policies, and cloud network parameters to understand their impact on network behavior. Together, these capabilities enable teams to conduct thorough testing across the board – from deploying new software and simulating security threats to optimizing resources and validating routing protocols – all without risk to the live network.
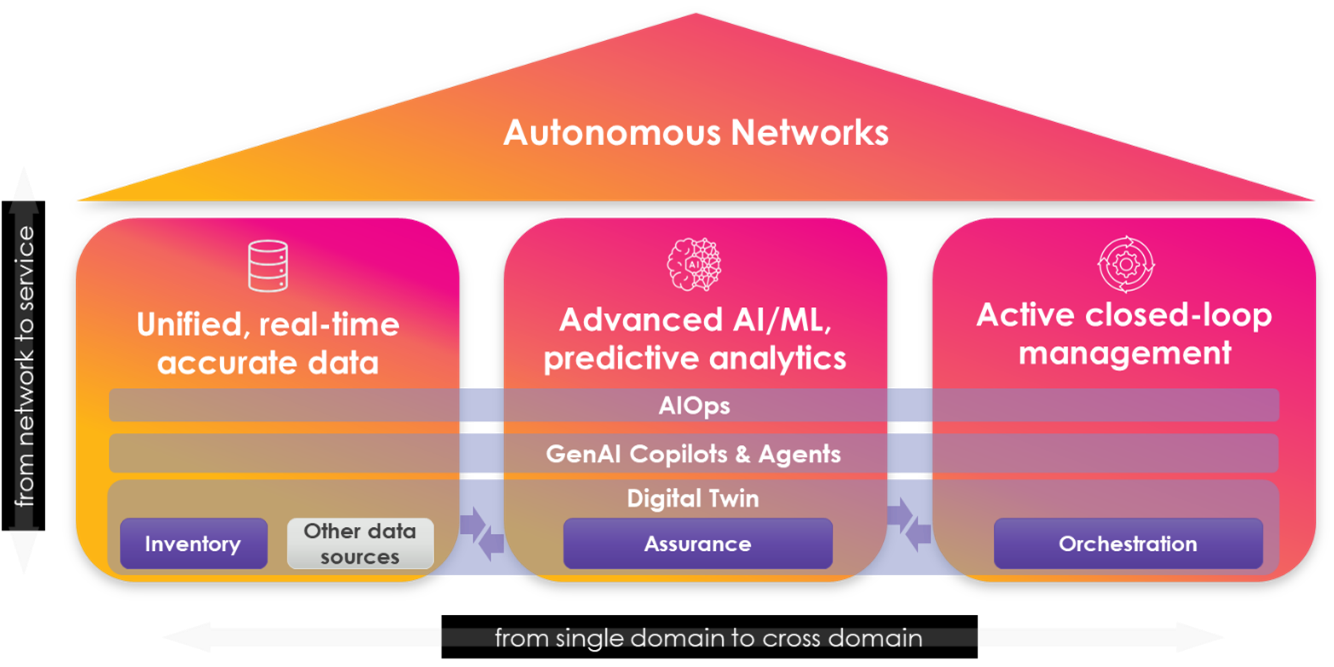
Figure 1 Key pillars in the Autonomous Networks Journey
Building intelligent tools
While a basic digital twin provides a real, or near real-time replica, AI integration transforms it into a dynamic and insightful tool. Specifically, AI algorithms provide the sandbox with intelligent capabilities that enhance its value for AN development. One key function is automated data analysis, whereby AI sifts through vast amounts of simulated network data, automatically identifying complex patterns, subtle anomalies, and potential areas for optimization that would be impossible via manual human effort. Predictive modeling is another important capability, enabling network behavior to be forecasted under a variety of conditions using historical and real-time data analysis. Examples include sudden traffic spikes or simulated equipment failures. Finally, AI facilitates resource optimization, where AI algorithms dynamically adjust network parameters such as bandwidth allocation, routing paths, and server load balancing to maximize efficiency based on simulated conditions.
In combination, these capabilities enable network engineers to proactively anticipate issues and implement solutions before they impact the network. So for example, they could predict link congestion based on simulated traffic patterns and automatically reroute traffic to avoid bottlenecks or optimize bandwidth allocation based on simulated user demand during peak hours.
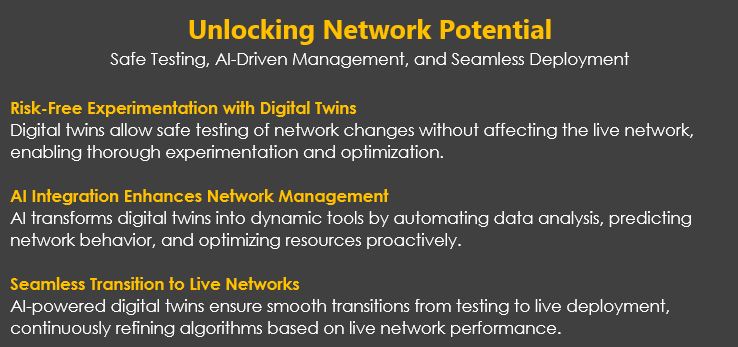
Transforming the sandbox into a dynamic learning environment
Powered by GenAI and autonomous agents, the digital twin becomes a truly dynamic learning environment. Agent-based AI introduces autonomous decision-making, allowing it to explore the simulated network environment and independently test different configurations and strategies. They then learn from the experiment outcomes, while adapting their behavior to achieve pre-defined goals such as minimizing latency or maximizing throughput. Crucially, these agents can also collaborate to solve complex network management challenges that would be too difficult for individual agents to handle.
Beyond agentic capabilities, GenAI brings powerful tools that allow it to automate scenario creation, generate complex and realistic network simulations that push AN algorithms to their limits, allowing the discovery of edge cases and vulnerabilities. It can also generate code for automation, producing scripts to implement solutions validated within the digital twin, significantly streamlining the transition to live network deployment. As an example, imagine a swarm of AI agents autonomously optimizing routing protocols in response to a simulated network outage or GenAI creating a highly realistic simulation of a 5G network slicing scenario with dynamically changing user demands.
A seamless transition
Beyond experimentation, the AI-powered digital twin enables smooth transitions to the live network by building confidence through extensive testing and validation of new AN algorithms and configurations. When operators test various scenarios and edge cases in this virtual environment, they minimize unexpected behavior during live deployment, leading to fewer disruptions and better user experience.
GenAI makes this transition even more efficient by automatically creating the code needed to implement validated solutions in the live network, reducing deployment time and potential errors. Then, after deployment, the digital twin continues learning from live network performance, creating a continuous feedback loop that refines algorithms and optimizes performance. The result is a true CI/CD environment, where networks adapt and improve while maintaining efficiency, resilience, and user satisfaction.
Leading the way in telco network management
Amdocs brings together solutions for digital twin implementation and intelligent network management, providing real-time visibility and insights into the network data layer. To achieve this, Network Inventory offers detailed tracking of network assets, while Service Assurance handles proactive monitoring and performance data. Amdocs E2ESO, part of our Intelligent Networking Suite, uses AI to optimize workload placement and network parameters to manage services throughout their lifecycle. Meanwhile, Amdocs amAIz, our AI & Data suite, leverages both AI and GenAI technologies to enhance predictive analytics and automation. Equipped with these capabilities, CSPs can confidently move from digital twin testing to live network deployment, ensuring continuous optimization of their ANs.